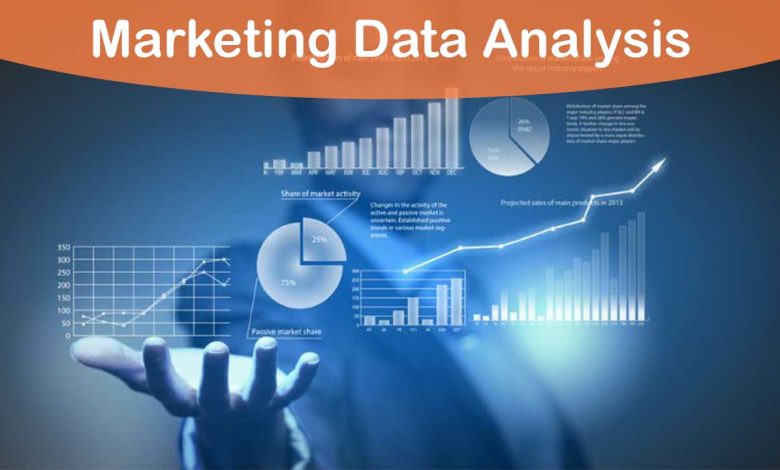
Data analysis in marketing involves evaluating large amounts of data to gain insights into customer behavior and market trends.
It includes steps such as data collection, exploratory data analysis, predictive modeling, and monitoring recommendations.
The ultimate aim is to make informed decisions, personalize marketing strategies, and drive business growth.
Marketing Data Analysis Steps
Data analysis in marketing plays a crucial role in driving business growth and making informed decisions.
The process of data analysis involves different steps starting from defining the marketing problem and research question, to implementing and monitoring recommendations.
The there 25 steps that I listed below, although not all of these steps are necessary but I listed those here to give you an idea for future analysis.
- Define the marketing problem and research question: Clearly define the problem you want to solve and the research question you want to answer. This will help guide the data collection and analysis process.
- Data collection: Gather data from various sources such as surveys, website analytics, social media analytics, and customer databases.
- Data pre-processing: Clean and pre-process the data to handle missing values, outliers, and ensure data quality.
- Exploratory Data Analysis (EDA): Use visual and statistical methods to understand and summarize the main characteristics of the data.
- Data visualization: Create visualizations to help identify patterns and relationships in the data.
- Segmentation analysis: Divide the data into subgroups based on common characteristics to understand how different segments behave and make decisions.
- Predictive modeling: Use statistical or machine learning models to make predictions about future customer behavior.
- Testing and validation: Test the models and validate the results using appropriate evaluation metrics.
- Insights and recommendations: Draw insights and make recommendations based on the findings.
- Implementation and monitoring: Implement the recommendations and monitor their impact on the marketing problem.
- Continuous improvement: Continuously monitor and evaluate the results and make adjustments as necessary to improve the analysis.
- Data integration: Combine data from various sources to get a comprehensive view of the customer and market. This
- Marketing Mix Modeling (MMM): Use econometric models to understand the impact of different marketing activities on sales and other business outcomes.
- Customer lifetime value (CLV) analysis: Analyze customer data to estimate the value of a customer over their lifetime and identify the most valuable segments.
- Churn analysis: Analyze customer data to identify customers who are at risk of leaving and develop strategies to retain them.
- Personalization: Use data and machine learning algorithms to personalize marketing messages and offers to individual customers.
- Marketing attribution: Use data and models to understand the impact of different marketing channels on customer behavior and measure the ROI of marketing activities.
- Competitive analysis: Analyze competitor data to understand their strengths and weaknesses, and identify opportunities for differentiation.
- A/B testing: Test different marketing strategies and tactics to determine the best approach for a particular market or customer segment.
- Predictive maintenance: Use machine learning algorithms to predict when equipment or products are likely to fail, allowing for proactive maintenance and reducing downtime.
- Sales forecasting: Use historical sales data and market trends to predict future sales and help plan production, staffing, and inventory.
- Consumer behavior analysis: Analyze customer behavior data to understand how customers make decisions and what drives their purchasing decisions.
- Market basket analysis: Analyze the items that customers purchase together to identify potential cross-sell and upsell opportunities.
- Social media analysis: Analyze social media data to understand customer sentiment, brand reputation, and the impact of marketing campaigns on social media.
- Customer feedback analysis: Analyze customer feedback data from surveys, customer service interactions, and other sources to understand customer needs and preferences.
Clean and Pre-process the Data
This step involves preparing the data for analysis by removing any errors, inconsistencies, and irrelevant information. The following are some common tasks in data cleaning and pre-processing:
- Handle missing values: Missing values can result from data collection errors or gaps in the data. Common techniques to handle missing values include imputing missing values, deleting rows or columns with missing values, or using statistical models to predict missing values.
- Remove outliers: Outliers are extreme values that can significantly affect the results of an analysis. Common techniques to remove outliers include removing rows with extreme values or transforming the data to minimize the impact of outliers.
- Handle Duplicates: Duplicate records can result from data entry errors or multiple sources of data. Duplicates should be identified and either removed or combined.
- Data normalization: Data normalization involves transforming the data into a common scale to ensure that all features are on the same scale. This can help prevent some machine learning algorithms from giving more importance to features with higher magnitudes.
- Data encoding: Categorical variables need to be encoded into numerical values in order to be used in statistical models. Common encoding techniques include one-hot encoding, label encoding, and ordinal encoding.
The goal of this step is to ensure that the data is of high quality and ready for further analysis. Clean and pre-processed data will lead to more accurate results and insights from the analysis.
Exploratory Data Analysis (EDA)
Exploratory Data Analysis (EDA) is the process of using visual and statistical methods to understand and summarize the main characteristics of a dataset. EDA is an important step in data analysis as it helps to identify patterns, relationships, and trends in the data, as well as uncover any potential errors or outliers.
The following are some common tasks in EDA:
- Summary statistics: Calculating descriptive statistics such as mean, median, mode, and standard deviation can provide a quick overview of the data distribution.
- Data visualization: Creating visualizations such as histograms, box plots, scatter plots, and heat maps can help to identify patterns and relationships in the data.
- Distribution analysis: Evaluating the distribution of the data can help to identify any skewness or outliers, and guide the choice of statistical models.
- Correlation analysis: Identifying the correlation between variables can help to determine which variables are related and may impact the results of the analysis.
- Hypothesis testing: Formulating hypotheses and testing them using statistical methods can help to determine the significance of relationships and patterns in the data.
EDA is an iterative process and may require multiple rounds of visualization and analysis to fully understand the data. The insights gained from EDA can be used to guide further analysis, inform the choice of statistical models, and provide a foundation for making data-driven decisions.
Visualize The Data
Data visualization is a crucial step in the data analysis process that allows marketers to make sense of large amounts of data and identify patterns and relationships in the data.
It involves creating graphical representations of data using tools such as bar charts, line graphs, scatter plots, and heat maps.
The steps involved in data visualization include:
- Determine the purpose of the visualization: What information do you want to communicate?
- Choose the appropriate visualization type: Select a visualization type based on the type of data and the information you want to convey.
- Prepare the data: Clean, format, and organize the data in a way that is suitable for visualization.
- Plot the data: Use a tool such as a bar chart, line graph, scatter plot, or heat map to create the visualization.
- Customize the visualization: Add labels, annotations, and formatting to make the visualization more clear and informative.
- Evaluate the visualization: Check the visualization for accuracy, readability, and effectiveness in communicating the intended message.
- Refine the visualization: Make changes to the visualization as needed to improve its effectiveness in communicating the information.
- Share the visualization: Share the visualization with others in a way that is accessible and easy to understand.
Segmentation Analysis
Segmentation is the process of dividing a target audience into smaller groups based on shared characteristics, behaviors, or demographics.
The goal of segmentation is to identify homogeneous groups of customers that can be targeted with tailored marketing messages and products.
Segmentation helps marketers to understand their customers’ needs and preferences, and to develop marketing strategies that are better suited to each segment’s unique needs.
To perform segmentation, data is collected from a variety of sources, such as surveys and customer interactions, and analyzed using statistical techniques, such as cluster analysis or decision trees.
The result of segmentation is a set of customer segments, each with its own profile, which can be used to inform targeted marketing strategies.
The steps involved in segmentation analysis include:
- Define the target audience: Identify the target audience you want to analyze and segment.
- Collect data: Gather relevant data from a variety of sources such as customer surveys, demographic data, and purchase history.
- Clean and pre-process the data: Clean, format, and prepare the data for analysis.
- Identify segmentation variables: Determine the variables that are most relevant for segmenting the target audience.
- Apply segmentation techniques: Use statistical techniques such as cluster analysis, factor analysis, or decision trees to segment the target audience into homogeneous groups.
- Evaluate the segments: Evaluate the segments based on their size, similarity, and overall quality.
- Select the best segments: Choose the segments that are most relevant and valuable for marketing purposes.
- Define segment profiles: Create profiles for each segment that describe their characteristics and behaviors.
- Analyze customer behavior: Analyze the behaviors and preferences of each segment in order to understand their needs and desires.
- Develop marketing strategies: Develop marketing strategies that are tailored to each segment’s needs and preferences.